
These platforms are not limited to industry but are supportive in various field such as service, assistance, teleoperation and also rehabilitation.
#CHALLENGE OF THE GOBOT SERIAL#
In order to increase the flexibility of such mobile platforms, it is also common practice to add serial manipulator arms to their yielding systems with nine degrees of freedom and more. Navigation, control and perception for mobile robots are vivid fields of research fostering advances in Industry 4.0. The organizers reserve the right to disqualify participants who are violating the rules or engage in scientific misconduct.Mobile robotics is already well established in today’s production lines.
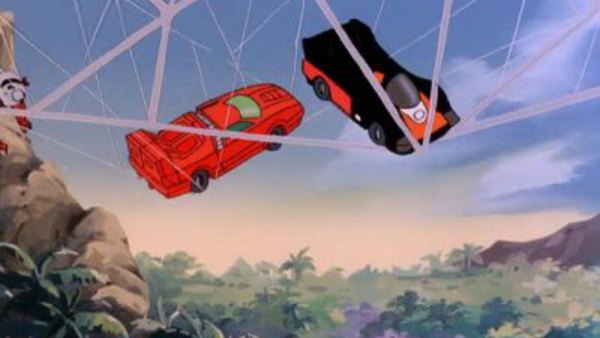
#CHALLENGE OF THE GOBOT CODE#
To be eligible to win prizes, participants agree to release their code under an OSI-approved license and to publish a report describing their method in a publicly accessible way (e.g.It is the responsibility of the team's contact person to distribute the prize money according to their team-internal agreements. Cash prizes will be paid out to an account specified by the contact person of each team.Each team needs to nominate a contact person and provide an email address through which they can be reached.Individuals are not allowed to participate in multiple teams.Participants may participate alone or in teams.It is not permitted to use data collected during evaluation rollouts or obtained from other sources.As an example, two prominent algorithmic approaches meeting this criteria are: offline reinforcement learning and imitation learning. Any algorithmic approach may be applied that learns the behavior only from the provided data and does not make use of any hard-coded/engineered behavior.
#CHALLENGE OF THE GOBOT HOW TO#
In the real robot stage, participants get access to dataset containing data collected on 6 robots.įor more detailed information on how to participate, please see the software documentation. In the pre-stage, participants only get access to datasets with data collected in simulation and also evaluate their policies only in simulation.
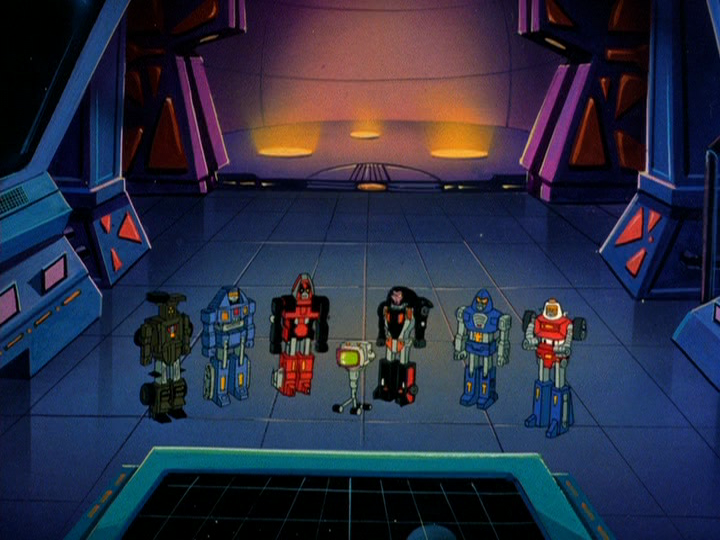
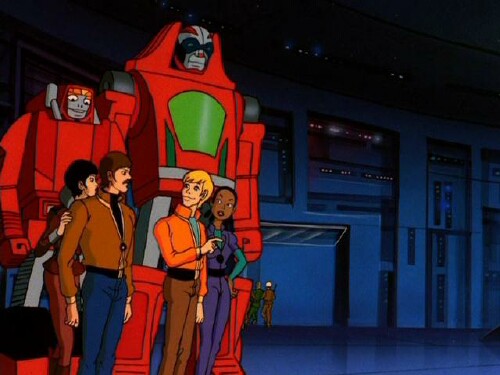
Push a cube to a target location on the ground.There are two tasks of different difficulty: The participants are provided with datasets containing dozens of hours of robotic data and can evaluate their policies locally in simulation (pre-stage) or remotely on a cluster of real TriFinger robots (robot stage). The goal of this year’s challenge is to solve dexterous manipulation tasks with offline reinforcement learning (RL) or imitation learning. For this the teams will get remote access to our TriFinger robot cluster. Robot Stage: The best teams of the pre-stage are admitted to the real-robot stage where the same tasks have to be solved on the real robots.This stage is done completely in simulation, so no access to the robots is needed.

